Let’s Drop the “AutoML vs. Data Scientist” Discussion
Rather than choosing to invest in either AutoML or data scientists, tech leaders must recognize that the future lies in both.
As technology leaders seek to apply machine learning to solve business problems, they must abandon the question of whether to invest in either automation or human data science talent, or risk losing the business value of both.
We have seen successful adoption of automation to manage infrastructure, and to apply continuous integration/continuous delivery (CI/CD) practices to reduce deployment timelines. In both cases, automation replaces manual processes that are tedious, time-consuming and error prone — increasing efficiency and freeing up human resources for more impactful work.
Automated machine learning (AutoML) promises to do exactly that for machine learning.
The tasks that AutoML tools perform are more elaborate, as machine learning is exponentially more complex than infrastructure or CI/CD. This is great news for technology leaders. Successfully automating a more intricate workflow means that businesses can reap higher rewards with less effort. As data scientist skill sets are expensive and difficult to come by, AutoML tools will enable organizations to access the benefits of machine learning solutions at more reasonable costs.
This has naturally prompted tech leaders and the data science community to compare AutoML to humans, asking which is better and whether data scientists will be left behind. But the answer to that question isn’t so clearly defined. Let’s examine why, and explore some other questions we should be asking instead.
Is AutoML better than a data scientist?
In the case of machine learning, the metric for “better” fluctuates based on the business problem you’re trying to solve.
AutoML tools have advantages over human data scientists in speed and risk reduction; but the human brain is superior to a machine in other ways. A data scientist brings a level of nuance, intuition and creative problem-solving to the process that AutoML simply cannot match.
Asking whether AutoML is better than human-built machine learning is like asking whether to rent a 3D printer or hire a sculptor with a master’s degree; the answer lies in what you need from the product.
Are you seeking a finely detailed, innovative creation that solves a new problem, or a mechanism that meets the correct dimensions and weight?
Like a 3D printer, AutoML tools can reach an acceptable level of accuracy in far less time than a human. If sufficient for the business use case, why not use AutoML rather than human hours? With automation, you relinquish full control in favor of efficiency; leaving you with limited ability to access and tweak the underlying models or algorithms for your solution. When the use case is more intricate, a human touch is crucial. A data scientist can create more-nuanced and higher-performing solutions for complex machine learning applications, such as feature engineering and model ensembling — while retaining complete control over the models and algorithms they build.
In other cases, the metric for “better” is not tied to results, but to the skills an organization has readily available. Not every company has a team of skilled data scientists, or a pressing need to invest in such a team. In these cases, AutoML can be labeled a “better” fit simply because it enables organizations to do more with the in-house skills they have.
Will AutoML replace data scientists?
The short answer is yes. We’re already seeing it happen.
In cases where a machine can build a machine learning model more efficiently and still achieve an acceptable range of accuracy, it makes sense for organizations to opt for AutoML. These tools open the door for developers without classical data science backgrounds to access machine learning. We’re seeing more software engineers developing machine learning knowledge on the job, forming a new contingent of “citizen data scientists.” They may not have the expertise to build complicated machine learning models, but they can leverage AutoML tools to solve real problems for their organizations.
While AutoML can carry some of the machine learning workflow without the need for data scientists, that doesn’t mean the data science skill set will become obsolete. Classically trained data scientists are just as important, if not more so, now that more organizations can access AutoML.
The “AutoML vs. data scientist” discussion is inherently flawed, and we encourage technology leaders to dive into the real question: How can businesses fully leverage AutoML and data scientists?
Data scientists are the real winners with AutoML
Smart tech leaders are quickly realizing that it’s not a matter of choosing either AutoML or data scientists, but of crafting a strategy to capitalize on both. AutoML will only increase the value of human data scientists.
The data scientists who embrace AutoML will be able to expand deeper into the capabilities of machine learning and become even more effective at what they do. In the time it would take a data scientist to handcraft ten experiments, they can now conduct a hundred experiments. Savvy data scientists see this as an opportunity to spend the bulk of their time exploring technical problems more exhaustively — bringing far bigger returns to their organizations.
Successful data scientists will embrace AutoML tools the way the construction industry embraces panelization and pre-fabrication tools: as a mechanism to reduce their time spent on repetitive tasks and allow a machine to prepare the materials they need to conduct more-specialized work.
Drop the AutoML vs. data scientist discussion — you will need both
It’s futile to try establishing a winner in the AutoML vs. data scientist argument. The future isn’t a discussion of AutoML or data scientists, it’s one of AutoML and data scientists.
The data scientists who embrace AutoML as an accelerator for their potential will be the real winners.
Technology leaders must embrace AutoML, not as a one-to-one replacement for a data scientist resource, but as a way to begin reaping the benefits of machine learning before you can build up an in-house data science skill set — and a way to propel the impact of in-house data scientists you do have. Astute organizations can start diving into AutoML right now, and with the support of a developer skill set, be better prepared to recruit top data scientist talent as they advance.

Tired of Navigating Tech Trends and Avoiding the Hype?
About the Authors
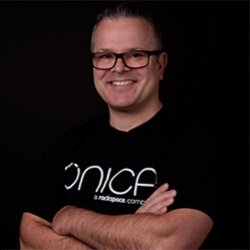
VP, Private Cloud Solutions
Eric Miller
An accomplished tech leader with 20 years of years of proven success in enterprise IT, Eric is a strong advocate of cloud native architectural patterns, passionate about Machine Learning, IoT, Serverless, and all things automation in the cloud. Eric has led several AWS and solutions architecture initiatives, including AWS Well Architected Framework (WAF) Assessment Partner Program, Amazon EC2 for Windows Server AWS Service Delivery Program, and a wide range of AWS rewrites for multi-billion dollar organizations. Prior to joining Rackspace, Eric was the Vice President of AWS Customer Solutions at Onica, which was acquired by Rackspace in 2019. Before working with Onica, Eric held several technology leadership positions at School Pointe, Inc., Neudesic, m2 Consultants, ARGUS International, Inc., Apex Mortgage Services LLC, and TechSkills. Eric lives in New Albany, Ohio with his wife and family. He holds a Bachelor of Science in Information Technology and Information Systems Security from the University of Phoenix.
Read more about Eric Miller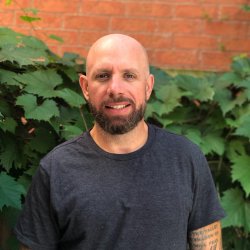
Practice Manager, Data Science & Engineering
Mark McQuade
Mark McQuade is an AWS and cloud-based solution specialist, knowledge addict and relationship builder. Earlier in his career, Mark held technical support, operations, business development and leadership roles for a telecommunications solutions provider, where he worked for 13 years. He then transitioned to the world of cloud and opened up his own AWS small business before joining Onica, who was acquired by Rackspace technology in 2019. Mark is currently Practice Manager of Data Science & Engineering at Rackspace Technology. Every day, he gets to learn more about what he is passionate about professionally – AI and machine learning – as well as the fascinating world of data. As a technology evangelist, you’ll often find Mark promoting data and AI/ML at talks, webinars, podcasts and industry events.
Read more about Mark McQuadeRelated Topics