The Evolution of Enterprise Data Supply Chains
Organizations need to recalibrate their data strategies to accommodate a growing supply chain of diverse data and utilize modern data architectures on which to build and maintain effective data supply chains.
With a growing supply chain of data, companies should refine how they approach data, re-evaluating data strategy and leveraging modern data architectures to build, use and maintain effective data supply chains.
Data has never been more essential to business, but many companies fall behind when it comes to addressing some common data challenges. With more access than ever to useful external data, many companies lack the processes to leverage outside information in a useful way. The focus remains on data infrastructure, rather than data products.
Let’s define “supply chains of data”
Imagine that data is similar to the raw materials used in manufacturing. In this analogy, raw data is being prepared for analysis – that is, you’re transforming it from raw material into a product. In this case, it’s moving from a raw state as part of source systems to a more refined state in order to feed advanced analytics and smart applications.
Whereas supply chain management tracks the movement of goods and services, supply chains of data track lineage, starting at the source of origination of the data. After data is extracted, it undergoes an enrichment process. By layering it across multiple sources of information, and cataloging and annotating it, data becomes available in a searchable format, wherein the end-user can access it, query it and put it to good business use. Finally, the data can be consumed as a data product, such as a financial data mart, customer or product 360, etc.
How do you manage all of this?
In the past, the data mostly came from operational data systems (ODS). This is data generated from within customer relationship management (CRM) and enterprise resource planning (ERP) systems or other products that serve businesses as transactional systems such as core products, workforce management, retail or point-of-sale systems, patient records, contracts, etc.
This used to be the predominant use of data: a handful of source systems ingested data into a central repository. Teams would then use that data as it became available within the large repository — often a data warehouse.
The evolution to multicloud and multi-modal supply chains of data
The use of data has changed in the last five to seven years, especially in the wake of the pandemic. It's no longer sufficient for a company to utilize only the data it generates internally. This is causing organizations to rethink their supply chains of data. Today, companies are considering two data supply models: direct and indirect. The direct model relies on internal and external third-party data sets, while the indirect model utilizes environmental factors, behaviors, influences and synthetic data sets.
- Direct:
- First-party data is collected directly through CRM, ERP, end-user devices and other internal systems of record.
- Second-party data is collected from partners, vendors and consumer devices.
- Third-party data, is collected from purchase histories, customer intelligence lists, market data, economic data, etc.
- Indirect:
- Causal data is a collection of data points that could enrich existing data models. For example, data related to external contributing factors, weather as an indicator for customer sentiment, demographics, social media, trends, etc.
- Synthetic data describes simulated and auto-generated data. For example, autonomous vehicle simulators need volumes of data that’s not readily available, and possible real world scenarios that have not been captured. Another key area being generative adversarial networks (GANs), in which a generative network generates data points while a discriminative network evaluates them to the point at which the data becomes indistinguishable from real world data points. This application is useful in privacy-constrained environments and AI models, where it can help to stem bias.
How should organizations adapt to this evolution?
The needs of the enterprise are much more interconnected today — not only within the company, but also among suppliers, producers and consumers. Organizations are swiftly adopting multicloud, with workload types as de-facto criteria for choosing a cloud. Applications are increasingly distributed across clouds, and the supply chain of data invariably trends toward multicloud.
The move to SaaS for business applications has also impacted reliability of your supply chain, which now extends outside your organization to third-party SaaS providers. The integration with a SaaS provider creates a push and pull model where direct data comes from the SaaS application, is enriched and master data is pushed back to the SaaS applications. For example, we know that retail sales typically decrease during periods of inclement weather. Integrating that data back into inventory controls is crucial to maintaining the stability and longevity of retail companies.
Data supply chain trends are also increasingly impacted by technology innovations that make data more easily available. This is where data-sharing concepts and easier access to curated data sets — both procured and open-source (a.k.a. data products) — come into play.
Data sharing is increasingly mainstream. This is where data is prepared by third-party companies, partners, suppliers, then made available through data-as-a-service vendors like Snowflake and Databricks. Internal data exchanges and marketplaces share these prepared data products across business units for increased velocity of collaboration.
While your supply chain may be exploding, in the case of data sharing, your supply chains are imploding because you're reducing the time and complexity of creating additional data pipelines. Instead of shipping data from one place to another and then integrating it, you can now transform your data without any data movement.
How do we take advantage?
Fifteen years ago, the data that an enterprise exploited lived entirely within that enterprise. Companies were quickly able to access data and create and leverage data points to create digital products.
Today, company data is distributed across multiple clouds and SaaS applications. Internal data alone isn't sufficient to enable better decisions. Instead, you’ll rely more on external data — information from your global partners, suppliers and consumers — and causal data that interprets the environment surrounding what you sell and who you sell to.
Considering these changes, how do you create architectures suitable for maintaining a growing supply chain of data? Here’s one starting point: Look carefully again at how your organization approaches its data strategy. You should account for the expanding supply chain of data to leverage all three forms of direct data sets (first-, second- and third-party data) and increasing the adoption of indirect data sets, such as causal and synthetic data. From there, you can build and leverage more modern data architectures and data integration patterns while maintaining a focus on data that is most critical to an enterprise.

Cybersecurity Annual Research Report 2022
About the Authors

Chief Architect - Data & AI
Nirmal Ranganathan
Nirmal Ranganathan is the Chief Architect – Data & AI at Rackspace Technology and responsible for technology strategy and roadmap for Rackspace's Public Cloud Data & AI solutions portfolio, working closely with customers, alliances and partners. Nirmal has worked with data over the past 2 decades, solving distributed systems challenges dealing with large volumes of data, being a customer advocate and helping customers solve their data challenges. Nirmal consults with customers around large-scale databases, data processing, data analytics and data warehousing in the cloud, providing solutions for innovative use cases across industries leveraging AI and Machine Learning.
Read more about Nirmal Ranganathan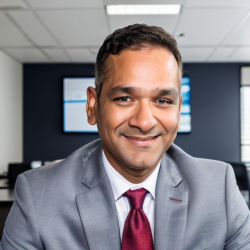
President AI, Technology and Sustainability
Srini Koushik
Srini serves as President AI, Technology and Sustainability at Rackspace Technology® and is responsible for technical strategy, product strategy, thought leadership and content marketing. Prior to joining Rackspace Technology, Srini was Vice President, GM, and Global Leader for Hybrid Cloud Advisory Services at IBM where he worked with CIOs on their hybrid cloud strategy and innovation. Before that, he was the Chief Information Officer for Magellan Health where helped double the company’s revenue in just four years. Prior to Magellan, he was the President and CEO of NTT Innovation Institute Inc., a Silicon Valley-based startup focused on building multi-sided platforms for digital businesses. Srini also serves on the advisory boards for Sierra Ventures, Mayfield Ventures and Clarigent Health. Srini is an innovative and dynamic executive with a track record of leading organizations to deliver meaningful business results through digital technologies, design thinking, agile methods, lean processes, and unique data-driven insights for the last two decades. Srini lives in Columbus, Ohio. When not working, he enjoys traveling around the world and learning to play the acoustic guitar.
Read more about Srini KoushikRelated Topics